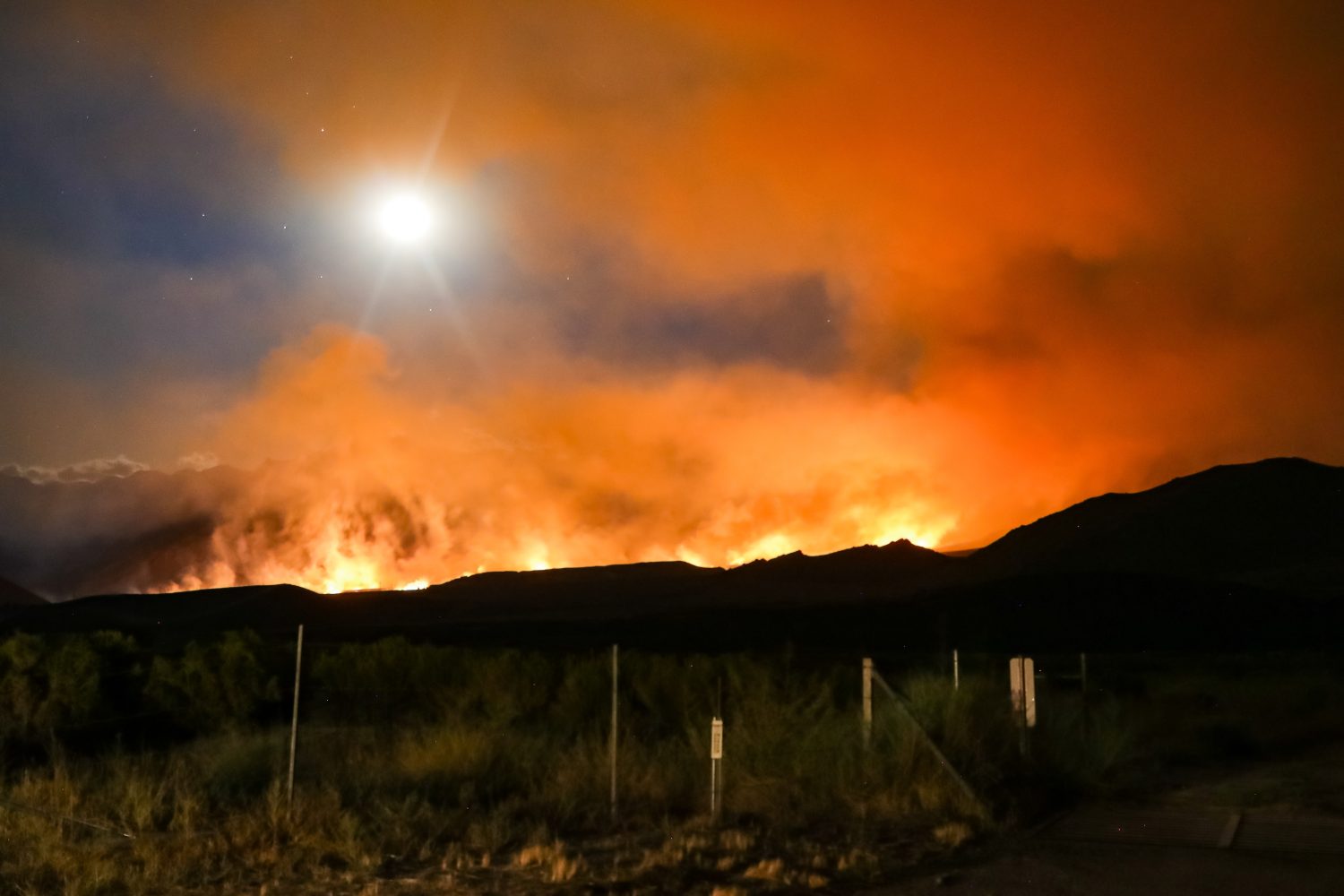
Over the past few years of terrible wildfires that have ravaged parts of Europe, Australia, and western US states, the critical use of UAVs in helping responders deploy to and battle hot spots has gotten deserved attention. Now, a group of researchers is developing a way of using deep learning neural computing applications analyzing drone images to automatically detect new fires, and identify hot spots requiring fastest reaction.
A group of professors at Indiana’s Purdue University has teamed up with computer and information technology graduate student Ziyang Tang to use neural networks and deep learning computers to speed up and strengthen the way images from drones can be used in fighting fires. At the moment, most analyses and decisions made based on aerial photos are taken by humans scouring footage taken over large expanses of burning land. The task is as huge as it is taxing, and the current objective is to make that faster and easier through automation.
Tang’s work began when he asked why the increasingly rapid and powerful interpretation and deduction capacities of computers weren’t being used to automate the search for wildfire for humans to act on.
“Deep learning has had great success with detecting objects like people and vehicles,” Tang said in an article published on Purdue’s news page. “But little has been done to help computers detect objects with amorphous and irregular shapes, such as spot fires.”
Tang and his academic partners went to work trying to harness the processing power that allows computers to detect drone-filmed objects with fixed sizes and regular shapes, and apply it to the rapidly changing and unpredictable forms of flames.
Reconstructing neural computer analysis of drone images to spot irregular, changing shapes of flames
The first step in that was introducing an algorithm that would identify flames, responders, and other factors involved in wildfire events captured in drone images and relay them to human monitors. The simplest, immediate goal was making the process of scanning so much footage taken over such vast areas less daunting and laborious through computerization.
“In an actual wildfire event, there is a distinct level of organized chaos,” Tang explained. “The operator of a UAS platform is often monitoring hundreds of spot fires and having to track multiple fire crews and related equipment on the ground. Because we are fallible and suffer from fatigue and short attention spans, some objects can be overlooked.”
The next task focused on overcoming two limitations in current neural network detection systems. First was changing their reliance on low-resolution images – usually 600 x 400 pixels – with an upgraded adaptation to the 4K footage that drones tend to take. As part of that, neural network detection methods that divide larger shots into many, equally sized boxes that are individually processed in search of catalogued images had to be changed. That procedure is not only time consuming, but risks missing fire that isn’t neatly limited to single quadrants.
In search of a solution, Tang and his team fed the 4K drone images of a controlled fire into their system. They created what they believe is the first public high-resolution wildfire dataset of 1,400 annotated photos containing 18,449 identifiable object like trucks, people, landscape features, and fire.
They also established a “coarse-to-fine” search approach to the automated detection of sparse, small, and irregularly shaped wildfire flames. In contrast to the analysis of each quadrant from photos that previous neural systems carried out, the course-to-fine method only scours boxes containing images of interest like probable flames – or sections in which such imagery overlaps two squares. The details of each of those is then passed along to human monitors much faster, since the process skips sections of the far bigger 4K superset unlikely to contain wildfire data.
“After extracting objects from high-resolution images, we zoom in to detect the small objects and fuse the final results back into the original images,” said Tang. “Our experiments show that the method can achieve high accuracy while maintaining fast speeds.”
FTC: We use income earning auto affiliate links. More.
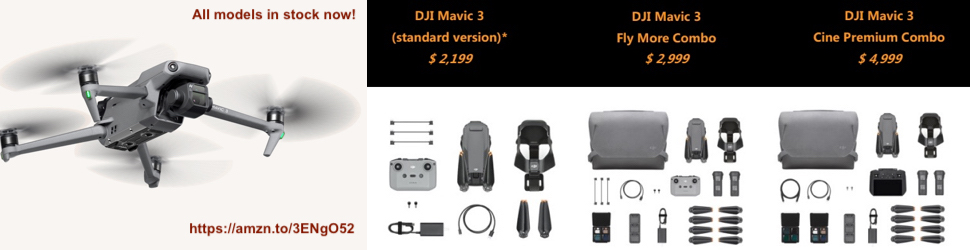
Comments